MCP vs. A2A Protocols: What Developers Need to Know About AI's New Plumbing
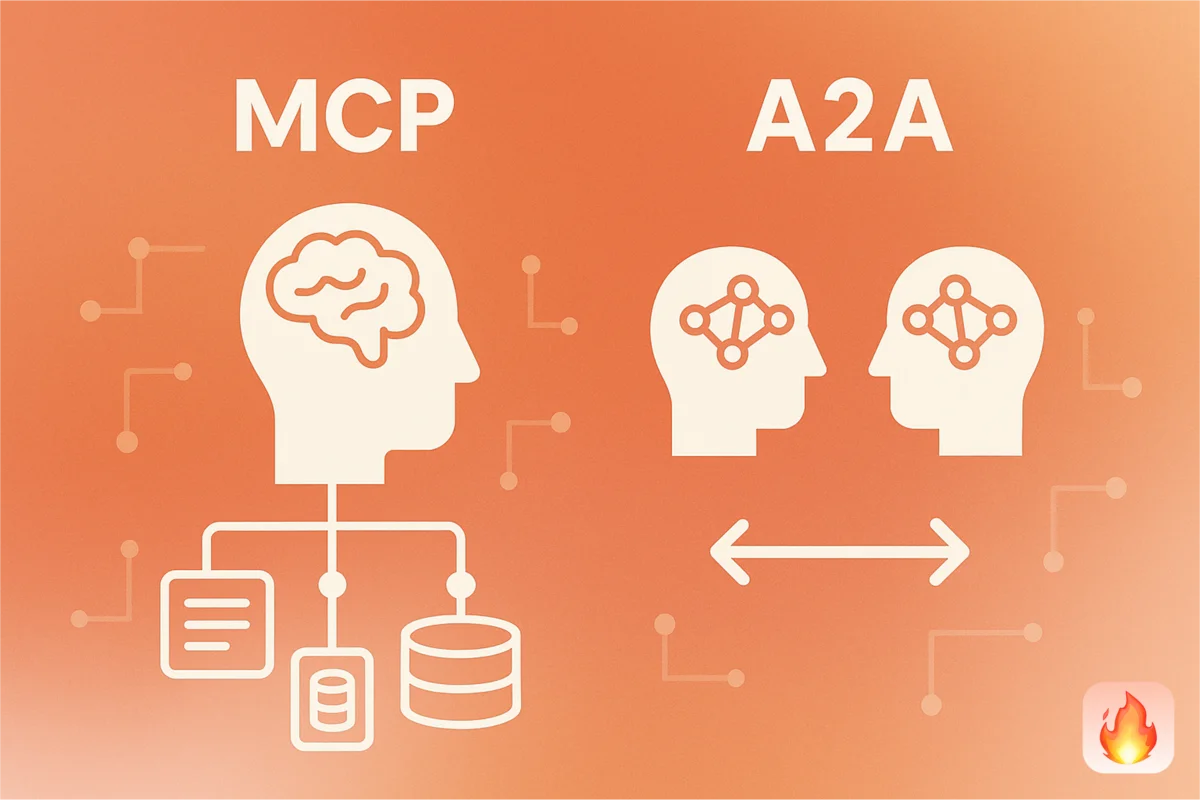
MCP vs. A2A Protocols: What Developers Need to Know About AI’s New Plumbing
We’re seeing AI models do remarkable things. They can write code, analyze data, and even hold surprisingly coherent conversations. But like a brilliant brain locked in a dark room, they’re often cut off from the real-time data, specialized tools, and importantly, each other. That’s starting to change.
Two new protocols are emerging as the potential standards for connecting AI to the outside world and enabling different AI systems to collaborate: the Model Context Protocol (MCP) and the Agent2Agent Protocol (A2A). They sound similar, and they share some technical roots, but they solve fundamentally different problems. Understanding the distinction is key for anyone building or using sophisticated AI applications.
So, what are they, and why should you care?
MCP: Giving AI Applications Access to the World
Imagine you’re using an AI-powered code editor. You want it to understand your entire codebase, not just the file you have open. Or maybe you want your chat assistant to pull live data from a specific database or trigger an external API. This is where MCP comes in.
Spearheaded initially by Anthropic, the Model Context Protocol (MCP) aims to be a standard way for LLM applications (like IDEs, chat interfaces – the ‘hosts’) to connect securely to external data sources and tools (the ‘servers’).
- Think of it like: The Language Server Protocol (LSP) standardized how code editors talk to language analysis tools (like linters or autocompleters). MCP aims to do the same for AI, standardizing how applications get context or access tools.
- How it works: It uses familiar JSON-RPC messages for communication between the host application and the MCP server.
- What it enables:
- Servers can provide
Resources
(files, database snippets, contextual information). For instance, an MCP server could use a service like Firecrawl to provide resources such as the real-time content of a specific webpage (even Firecrawl’s own homepage!) or structured data extracted from it. - Servers can offer
Prompts
(pre-defined workflows or templates). - Servers can expose
Tools
(specific functions the AI can be instructed to execute).
- Servers can provide
- Key Idea: MCP is about enriching a single AI application by giving it controlled access to external capabilities and data, with a strong emphasis on user consent for security.
A2A: Enabling AI Agents to Collaborate
Now, consider a more complex task: planning a multi-city business trip. This might involve searching for flights, finding hotels that meet certain criteria, checking calendar availability, and booking everything. You could imagine different specialized AI agents handling each part: a flight-search agent, a hotel-booking agent, a calendar agent. How do they work together? That’s the problem Agent2Agent (A2A) tackles.
Driven by Google and a consortium of partners, A2A focuses on enabling different AI agents, potentially built by different vendors using different frameworks, to communicate, coordinate, and collaborate on tasks.
- Think of it like: A universal translator and rulebook for meetings between different AI agents.
- How it works: It also builds on standards like HTTP, SSE, and JSON-RPC. It defines interactions between a ‘client’ agent initiating a task and a ‘remote’ agent acting upon it.
- What it enables:
Capability Discovery
: Agents can advertise what they can do via an “Agent Card”.Task Management
: A standardized “task” object allows agents to track the status of collaborative work, even for long-running processes.Collaboration
: Agents can exchange messages containing context, instructions, results (Artifacts
), etc.User Experience Negotiation
: Agents can figure out the best way to display information (text, forms, even video).Modality Agnostic
: Designed to handle more than just text (audio, video).
- Key Idea: A2A is about creating a multi-agent ecosystem where specialized agents can work together to achieve complex goals.
The Crucial Difference: App-to-Tool vs. Agent-to-Agent
The core distinction is simple:
- MCP: Connects one application to external resources/tools. (App → Tool Server)
- A2A: Connects multiple agents to each other for collaboration. (Agent ↔ Agent)
They aren’t rivals; they are designed to be complementary. An agent involved in an A2A collaboration might very well use an MCP connection to fetch data or execute a specific tool needed for its part of the task. MCP provides the resource, A2A provides the framework for collaboration using potentially many such resources.
Why This Matters
For developers and businesses, these protocols represent the essential plumbing for the next wave of AI.
- MCP lowers the barrier to creating AI applications that are deeply integrated with real-world data and specific functionalities, moving beyond generic knowledge.
- A2A paves the way for automating complex, multi-step processes by allowing specialized AI agents to work in concert, creating workflows that are more powerful than any single agent could manage alone.
Instead of countless bespoke integrations, MCP and A2A offer the promise of standardized connections. They move us from isolated AI brains to interconnected systems, capable of more complex and useful work. Watching how these protocols evolve and gain adoption will be crucial for understanding the future of practical AI implementation.
Learn More:
- MCP Specification: https://modelcontextprotocol.io/specification/2025-03-26
- A2A Specification & Blog: https://github.com/google/A2A, https://developers.googleblog.com/en/a2a-a-new-era-of-agent-interoperability/
P.S. Need to power your AI applications with reliable, up-to-date web data or build integrations like the ones described? Check out Firecrawl for powerful scraping, crawling, and data extraction APIs.
About the Author
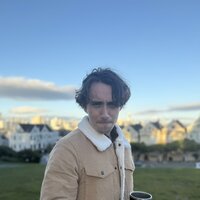
Caleb Peffer is the Chief Executive Officer (CEO) of Firecrawl. Previously, built and scaled Mendable, an innovative "chat with your documents" application, and sold it to major customers like Snapchat, Coinbase, and MongoDB. Also co-founded SideGuide, a tool for learning code within VS Code with 50,000 users. Caleb has a passion for building products that help people do their best work. Caleb studied Computer Science and has over 10 years of experience in software engineering.
More articles by Caleb Peffer
Using LLM Extraction for Customer Insights
Using LLM Extraction for Insights and Lead Generation using Make and Firecrawl.
MCP vs. A2A Protocols: What Developers Need to Know About AI's New Plumbing
Understanding the distinction between Model Context Protocol (MCP) and Agent2Agent Protocol (A2A) - two emerging standards for connecting AI to the outside world and enabling AI systems to collaborate.